The Argonne Leadership Computing Facility (ALCF) is building a testbed comprised of advanced artificial intelligence (AI) platforms. Designed to explore the possibilities of high-performance computing (HPC) architectures, the ALCF AI Testbed will enable the facility and its user community to help define the role of AI accelerators in next-generation scientific machine learning.
“The testbed combines a number of bleeding-edge components, including the Cerebras CS-2, a Graphcore Colossus GC22, a SambaNova DataScale system, a Groq system, and a Habana Gaudi system,” Venkatram Vishwanath, lead for ALCF’s Data Science Group. “The juxtaposition of these machines is unique to the ALCF, opening the door to AI-driven data science workflows that, for the time being, are effectively unfeasible elsewhere. The AI testbed also provides a unique opportunity for AI vendors to target their system—in terms of both software and hardware—to meet the requirements of scientific AI workloads.”
The Cerebras CS-2 is a wafer-scale deep learning accelerator comprising 850,000 processing cores, each providing 48KB of dedicated SRAM memory for an on-chip total of 40GB and interconnected to optimize bandwidth and latency. Its software platform integrates popular machine learning frameworks such as TensorFlow and PyTorch.
The Graphcore Colossus, designed to provide state-of-the-art performance for training and inference workloads, consists of 1,216 IPU tiles, each of which has an independent core and tightly coupled memory. The Dell DSS8440, the first Graphcore IPU server, features 8 dual-IPU C2 PCIe cards, all connected with IPU-Link technology in an industry standard 4U server for AI training and inference workloads. The server has two sockets, each with 20 cores and 768GB of memory.
The SambaNova DataScale system is architected around the next-generation Reconfigurable Dataflow Unit (RDU) processor for optimal dataflow processing and acceleration. The SambaNova is a half-rack system consisting of two nodes, each of which features eight RDUs interconnected to enable model and data parallelism. SambaFlow, its software stack, extracts, optimizes, and maps dataflow graphs to the RDUs from standard machine learning frameworks, including TensorFlow and PyTorch.
A Groq Tensor Streaming Processor (TSP) provides a scalable, programmable processing core and memory building block able to achieve 250 TFlops in FP16 and 1 PetaOp/s in INT8 performance. The Groq accelerators are PCIe gen4-based, and multiple accelerators on a single node can be interconnected via a proprietary chip-to-chip interconnect to enable larger models and data parallelism.
The Habana Gaudi processor features eight fully programmable VLIW SIMD tensor processor cores, integrating ten 100 GbE ports of RDMA over Converged Ethernet (RoCE) into each processor chip to efficiently scale training. The Gaudi system consists of two HLS-1H nodes, each with four Gaudi HL-205 cards. The software stack comprises the SynapseAI stack and provides support for TensorFlow and PyTorch.
Available to the research community beginning in early 2022, the ALCF testbed’s innovative AI platforms will complement Argonne’s next-generation graphics processing unit- (GPU-) accelerated supercomputers, the 44-petaflop Polaris system and the exascale-class Aurora machine, to provide a state-of-the-art computing environment that supports pioneering research at the intersection of AI and HPC.
“The last year and a half have seen Argonne collaborate with a variety of AI-accelerator startups to study the scientific applications these sorts of processors might be used for,” ALCF Director Michael Papka said. “GPUs have an established place in the future of scientific HPC, one cemented by years of dedicated research and well-represented in the first generation of exascale machines. It seems clear that AI accelerators could play just as prominent and expansive a role as GPUs, but the specifics of that role largely have yet to be determined. The scientific community should be active in that determination.”
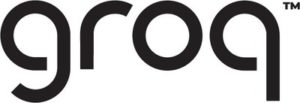
Groq logo
“The possibilities of ways to approach computing are constantly multiplying, and we want our users to be able to identify the most appropriate workflow for each project and take full advantage of them so as to produce the best possible science and accelerate the rate of discovery,” added Papka.
Offering architectural features designed to support AI and data-centric workloads, the testbed is uniquely well-suited to handle the data produced by large-scale simulation and learning projects, as well as by light sources, telescopes, particle accelerators, and other experimental facilities. Moreover, the testbed components stand to significantly broaden analytic and processing abilities in the project workflows deployed at the ALCF beyond those supported by traditional CPU- and GPU-based machines.
The ALCF testbed also opens the door to further collaborations with Argonne’s Data Science and Learning, Mathematics and Computer Science, and Computational Science Divisions, as well as the broader laboratory community. Such collaborations are of great utility, as they simultaneously deepen scientific discovery while validating and establishing the capabilities of new hardware and software through the use of real data.
The extensive, diverse collaboration with startups is essential for determining how AI accelerators can be applied to scientific research.
“The AI testbed, compared to our other production machines, feels much more like the Wild West,” Papka explained. “It’s very much geared for early adopters and the more adventurously inclined among our user community. This is facility hardware at its most experimental, so while we will certainly stabilize things as much as possible and provide documentation, it’s going to represent an extreme end of the scientific-computing spectrum.”
Nonetheless, a series of DOE-wide town hall meetings over the last two years has helped foster interest in the possibilities of AI for science, inviting a wealth of different perspectives and culminating in an extensive report that highlights the challenges and opportunities of using AI in scientific research.
Diverse Applications
Testbed applications already range from COVID-19 research to multiphysics simulations of massive stars to predicting cancer treatments.
Pandemic research is using AI technologies to address the fundamental biological mechanisms of the SARS-CoV-2 virus and associated COVID-19 disease, while simultaneously targeting the entire viral proteome to identify potential therapeutics.
The CANDLE project, meanwhile, attempts to solve large-scale machine learning problems for three cancer-related pilot applications: predicting drug interactions, predicting the state of molecular dynamics simulations, and predicting cancer phenotypes and treatment trajectories from patient documents.
Over the next year, the ALCF will continue to ramp up production use for the AI testbed, thereby solidifying its unique position among the facility’s powerful computing resources.
“The initial projects underway are just the tip of the iceberg,” Papka said. “The machines comprising the testbed will soon be leveraged for work that touches on virtually every discipline. While we don’t know exactly what’s going to happen, these systems will have an important role in shaping the scientific computing landscape.”
source: Nils Heinonen