By Timothy Sherbak, Quantum Corp.
From democratizing coding to amplifying productivity, AI has extraordinary potential to streamline our lives. But it also poses challenges for IT organizations that support AI initiatives: one, a proper infrastructure to support AI workloads that require extremely high compute and storage performance, and two, a secondary storage infrastructure to retain the massive amount of data necessary for both today’s and “tomorrow’s” AI model training. Increasing amounts of data must be stored and protected at low cost, and at the same time, it must be easily accessible to mine the insights within it.
For businesses, there’s the specific problem of having an ever-growing amount of data to contend with while also wanting to retain it for future analysis opportunities. Before there was widespread AI usage, massive data sets mainly existed in HPC environments. However, this infrastructure challenge is faced by organizations that need to capitalize on AI. There’s a lot these organizations can learn from HPC.
The storage needs for organizations working to exploit AI have now bifurcated. While high performance solid-state storage is essential to power AI workloads and deliver real-time analytics and processing using “hot” data, there’s also the need to store, protect and retain massive data sets for extended periods of time and make them available for analysis on a random, but semi-regular, basis. Sound familiar, HPC community?
The Evolution of Data
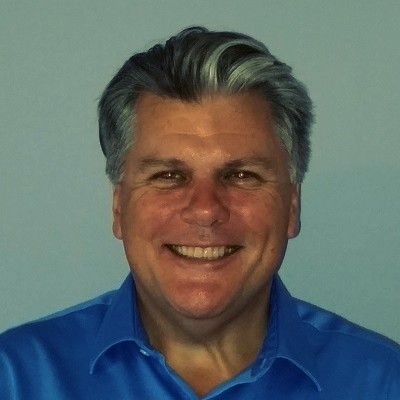
Timothy Sherbak, Quantum
The question organizations should ask is no longer “what data do I have,” but rather, “what data do I need” for analysis, repurposing and AI model training. It’s critical to architect a solution that simplifies searching massive data stores, enables organizations to index, tag and catalog data, making it easy to find, enrich and repurpose the data they need for AI.
This applies to every kind of organization. Think of a sports organization that has endless hours of footage taken over decades. Intelligently tagging, cataloging, and indexing their video assets enables them to easily search and find a clip they need from across their archives for a highlight reel or any other purpose.
Organizations should adopt data storage and management capabilities that can scale along with their different needs. It’s critical that organizations employ a complete solution that covers the entire lifecycle of data, delivering the performance required for AI workloads and immediate analysis, along with an effective way to move that data easily to a lower-cost, secure solution to retain that data.
For instance, your data catalog should be sitting in solid-state storage to quickly search and track down relevant data, while the data itself resides in an online storage archive (for example, an object store) that relies on low-cost media, such as tape. Simple tools and protocols provide for easy retrieval and staging of data from the archive to a high-performance analysis cluster based on GPUs and NVME storage. The combination of archives coupled with analysis clusters provide an affordable way to expand the use of AI and deep learning.
For archival storage, tape continues to be the preferred medium due to its maturity, low-cost, low power consumption and durability. Yet, new technologies are evolving the way tape is deployed and used, providing for greater simplicity and massive scale. Software-defined storage solutions based on RESTful APIs and simple browsers provide transparent access to massive amounts of data with interactive, self-service tools providing transparent access to tape-based data. RAIL (Redundant Array of Independent Libraries) architectures based on multi-dimensional erasure coding technologies enable highly available, fast access to these resources across multiple sites.
Navigating New Storage Strategies
The rapid proliferation of AI technologies has underscored the importance of being ready and quickly adapting to “what’s next.” Taking a cue from classic HPC environments, this approach not only caters to present requirements but also possesses the ability to scale and flex to accommodate unpredictable demands in the future. With modern data lifecycle management tools and technologies in place, AI infrastructure will start to mimic HPC environments—but with a whole new generation of underlying technology.
Timothy Sherbak is Enterprise Products and Solutions at Quantum Corp.