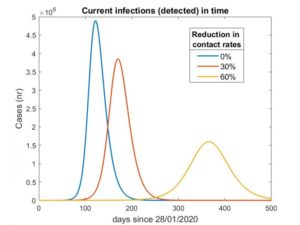
Different scenarios based on a reduction of contacts by 0, 30%, and 60%. This plot shows that the curve becomes flatter and wider as the number of contacts is reduced.
Credit: Barbarossa, et al. DOI: 10.1101/2020.04.08.20056630
GCS Centres in Germany are providing essential HPC tools for drug discovery and epidemiological modeling in the fight against the global pandemic
In December 2019, the world learned of a new and deadly pathogen. News coming out of Wuhan, China confirmed public health experts’ worst fears–a novel coronavirus appeared to have jumped from animals to humans. It was extremely contagious, and its penchant for hospitalising and killing vulnerable individuals has led to sweeping and indefinite changes to daily life around the globe.
Molecular biologists, chemists, and epidemiologists responded quickly in a race to combat the pandemic. As the full extent of the threat became clear in early March, the Gauss Centre for Supercomputing (GCS) joined the effort, announcing that it would fast-track applications for computing time aimed at stopping the spread of the virus or developing new treatments. Since then, GCS has supported roughly a dozen projects focused on epidemiological and drug discovery research, and remains committed to supporting scientists around the globe who are working tirelessly to combat the world’s worst pandemic in at least a generation.
Coronaviruses are a broad class of virus that cause illnesses ranging from the common cold to the severe acute respiratory syndrome (SARS) illness that first appeared in humans at the turn of the century. The pandemic coursing across the world over the last 6 months is also a coronavirus, known as SARS-CoV-2, which causes the illness ‘coronavirus disease 2019′ (COVID-19). As of May, 2020, the world has no proven course of treatment, and promising vaccine candidates are just beginning human trials.
Coronavirus spreads when droplets of infected individuals’ saliva are transmitted by coughing, sneezing, or speaking to other individuals, who absorb them through the mucous membranes of the nose and mouth. Although evidence is not conclusive, the virus might also spread through contact with infected saliva droplets that land on surfaces. While medical experts largely understand how the virus spreads, humans have no effective immunity against emerging diseases stemming from novel viral strains like SARS-CoV-2. This means that containment and social isolation are the most effective tools for buying researchers time to study treatments, develop vaccines, and create tools for tracking disease spread.
While societies have shuttered businesses and populations have largely remained at home, scientists are doing everything possible to support medical professionals at the front lines of the pandemic. Computational biologists and chemists have been using high-performance computing (HPC) to understand the virus at a molecular level, in order to identify potential treatments and accelerate the development of an effective vaccine. Epidemiologists have turned to the power of supercomputers to model and predict how the disease spreads at local and regional levels in hopes of forecasting potential new hot spots and guiding policy makers’ decisions in containing the disease’s spread. GCS is supporting several projects focused on these goals.
Searching for the next outbreak: epidemiological modeing to track COVID-19
While researchers begin to understand how coronavirus spreadson a person-to-person level, modelling how it spreads in communities or regions requires significant amounts of computing power and access to quality data. Even before Germany began seeing its first COVID-19 cases, leadership at the Jülich Supercomputing Centre (JSC) started collaborating with researchers at the University of Heidelberg and the Frankfurt Institute for Advanced Studies (FIAS) who had been modelling the disease’s spread in China. JSC offered its computational tools and expertise to digitalise epidemiological modelling and ultimately help predict how the virus would spread at state and local levels in Germany.
At the very beginning of this crisis, we were interested in how we could support early reaction and detection systems like computational scientists can do with tsunami or earthquake simulations,” said Prof. Thomas Lippert, Director of JSC. “As this is a very dynamic situation, we began to model system changes and we try to predict developments.”
With the pandemic still actively spreading around the globe, researchers knew that running quantitative, retrospective analyses of the situation was not yet appropriate. However, supercomputers could be used to combine datasets on infection growth rate, the so-called reproduction number (Rt), and the virus incubation time to create predictive models. With supercomputers, researchers begin to run suites of scenarios to predict the mortality rate on a local and national level based on the degree of social distancing measures and other actions meant to slow the virus’s spread
“The qualitative validity of these models comes from the fact that one can play through the different assumptions and detailed interactions, so you can validate those methods with hard data,” Lippert said. “Then you put these different measures into the model and see what it is doing. We can then ask, ‘when we put these measures together, are they moving things in a positive or negative direction?'”
Lippert noted that such models became less accurate the farther into the future they tried to model, but that their early results were accurate enough to help guide policy makers.
In a paper we published based on data up to March 20, we predicted the situation in Germany for April 20 within a few percent,” he said. “Because we already knew what measures were in place around the country, our work was pretty good at these predictions. Nevertheless, the model still underestimated the number of fatalities. At the policy and public health level, that means that if our data seems to overestimate the number of fatalities, it may not actually be doing that.”
Lippert, JSC researchers Dr. Jan Meinke, Dr. Jan Fuhrmann, and Dr. Stefan Krieg, and principal investigator and University of Heidelberg / FIAS research group leader Dr. Maria Vittoria Barbarossa were all contributors to a position paper published April 13 by the Helmholtz Association of German Research Centres. The paper, which was signed by the leadership of the Helmholtz Association and co-authored by 17 researchers, presented 3 scenarios for German government officials with respect to easing restrictions imposed during the COVID-19 pandemic.
The team demonstrated that if contact restrictions were raised too quickly, the Rt value would quickly rise over 1 (an Rt value of 1 representes that each infection will spawn 1 new infection), and Germany’s healthcare system could become overburdened within several months. In the second scenario, researchers modelled easing restrictions gradually and adopting an aggressive “feedback-based” contact tracing model to help slow the disease spread throughout the country. While in principle this scenario seemed promising, it required that significant contact restrictions would remain in place for an extended period of time–think months rather than weeks. The third scenario had the most resonance with German policy makers–keeping strong contact restrictions in place several weeks longer to help the Rt drop well below 1, then beginning the gradual reopening process.
International collaborations converge on essential drug discovery work
While predicting the virus’s spread over the initial weeks and months of the pandemic is essential, making it possible for society to return to normal will require development of effective treatments and scalable vaccinations to protect from infection.
University College London (UCL) Professor Dr. Peter Coveney has long leveraged supercomputers to understand drug interactions with pathogens and the human body. Since 2016, he has led the European Union’s Horizon 2020-funded project CompBioMed, which stands for ‘Computational Biomedicine,’ and its successor project, CompBioMed2. Both projects focus on accelerating drug discovery by augmenting experimental validation with modelling and simulation.
In the face of the COVID-19 pandemic, Coveney and over a hundred of his peers jumped into action, in part focusing their knowledge and access to HPC resources on indentifying existing drug compounds that could turn the tide against the virus. Specifically, Coveney and his collaborators model the binding affinities of drug compounds and pathogens. A drug’s binding affinity essentially means the strength of the interaction between, for instance, a protein in the lifecyle of a virus and active compounds in a medication–the stronger the binding affinity, the more effective the drug.
We can compute binding affinities in a matter of hours on a supercomputer; the size of such machines means that we can reach the industrial scale of demand necessary to impact drug repurposing programs,” Coveney said. “This can save us enormous amounts of wall-clock time and resources, including person hours, which are very precious in such a crisis situation.”
Supercomputers allow researchers to run large numbers of binding affinity simulations in parallel. Here, they compare information about the structure of the virus with a database containing information about known drug compounds to identify those with a high likelihood of binding. This computational approach enables researchers to investigate large numbers of potential drugs much more quickly than would be possible if they had to mix individual drug samples with actual viruses in a lab. Coveney has been using the SuperMUC-NG supercomputer at the Leibniz Supercomputing Centre (LRZ) to run many of his binding calculations.
SuperMUC-NG offers us an immense capability for performing a large number of binding affinity calculations using our precise, accurate and reproducible workflows–ESMACS (Enhanced Sampling of Molecular dynamics with Approximation of Continuum Solvent) and TIES (Thermodynamic Integration with Enhanced Sampling),” Coveney said. “So far, we have already performed a few hundred such calculations very quickly.”
Coveney has long collaborated with LRZ, developing his workflow to scale effectively on multiple generations of the SuperMUC architectures. LRZ Director Prof. Dieter Kranzlmüller saw the recent work as a continuation of Coveney’s efforts. “Our long-term collaboration has enabled us to immediately identify and reach out to Peter to offer our assistance,” he said. “By strongly supporting research in drug discovery activites for years, we were in a position to ensure that research toward identifying therapeutics could be accelerated right away.”
Coveney has been performing his work as part of the Consortium on Coronavirus, an international effort involving researchers and resources from 9 universities, 5 United States Department of Energy national laboratories, and some of the world’s fastest supercomputers, including SuperMUC-NG (currently number 9 in the Top500 list) and Summit at Oak Ridge National Laboratory in the United States (currently the world’s fastest machine for open science). “This consortium is a vast effort, involving many people, supercomputers, synchrotron sources for experimental structural biology and protein structure determination, wet labs for assays, and synthetic chemists who can make new compounds,” Coveney said. “In all, it’s a massive ‘one-stop shop’ to help fight COVID-19.”
Considering the team’s ability to use supercomputers to run many iterations of drug binding affinity calculations, Coveney, who leads the European side of the consortium, is grateful for as much access access to world-leading supercomputers as he can get. “Our workflows are perfectly scalable in the sense that the number of calculations we can perform is directly proportional to the number of cores available,” he said. “Thus, having access to multiple HPC systems speeds things up substantially for us. Time is of the essence right now.”
With access to HPC resources in Europe and the United States, Coveney and his collaborators have narrowed a list of several hundred drug compounds and identified several dozen that have the potential to inhibit SARS-CoV-2’s replication in the body. In total, Coveney and his colleagues have scanned millions to billions of possible compounds via machine learning, ultimately helping them narrow down existing and novel compounds to find the most promising candidates. Once machine learning helps identify the most promising candidates, these are then subjected to computationally intensive, physics-based simulations, which provide more accurate calculations.
Molecules in motion: molecular dynamics simulations for observing drug-virus interactions
As a traditional leader in computational engineering, the High-Performance Computing Center Stuttgart (HLRS) staff has extensive experience supporting molecular dynamics (MD) simulations. In the realm of engineering, MD allows researchers to understand how combustion processes happen from the moment of ignition, but in the realm of computational biology, researchers can turn to these computationally intensive simulations to investigate how molecular structures in proteins move and interact at extremely high resolution.
A team led by Prof. Dr. José Antonio Encinar Hidalgo at the Universidad Miguel Hernandez in Elche, Spain has been using HPC resources at HLRS to run molecular dynamics simulations and molecular docking models for 9,000 different drug candidates to fight COVID-19.
Proteins on human cells and viruses come in distinctive shapes, and designing effective treatments requires that researchers understand the molecular configurations most likely to bind to one another. Molecular docking simulations serve as a basis for determining drug binding affinities–by simulating the sturctures of panels of drug compounds in various molecular positions, researchers can assess their potential to bind to and inhibit the function of viral proteins.
Encinar noted that while some molecular docking simulations could be performed on more modest computing resources, HLRS’s supercomputer enabled the team to put these snapshots of molecular docking configurations in motion through the use of molecular dynamics simulations.
Our calculations consisted of some 90 molecular dynamics simulations,” Encinar said. “On Hawk, a simulation takes approximately 5 days to calculate. But Hawk also enables us to calculate about 50 simulations at a time. In two weeks, we have all the necessary data. This work is not approachable in adequate time without high-performance computing resources.”
The team just published a journal article demonstrating its work scanning of 9,000 different drug compounds. It identified roughly 34 candidates that appear to have a high likelihood of inhibiting one of the key proteins of SARS-CoV-2.
Dreams of vaccines and hope for the future
In addition to the work described above, dozens of researchers focusing on other aspects of drug discovery and epidemiology related to COVID-19 have been granted access to HPC resources at the GCS centres through GCS’s fast-track program as well as PRACE’s calls for fast-tracked access to Europe’s leading HPC resources. (For a full list of COVID-19 related projects running at the GCS centres, click here).
The ultimate goal for scientists, medical professionals, and government officials, though, lies in developing an effective vaccine and scaling up production on a global scale. Coveney indicated that supercomputers have already helped pave the way for vaccine trials, enabling researchers to comb through 30,000 DNA sequences and to design vaccine candidates that are currently entering the testing phase. There are some aspects of fighting a pandemic that supercomputing cannot accelerate, though, and as vaccine candidates enter clinical trials, societies around the globe can only hope that the foundational work done by computational scientists has helped make identifying and designing a vaccine as efficient as possible.
Coveney was encouraged by the degree of collaboration we are currently witnessing between researchers across the globe. “Drug design involves a long and tedious pipeline of tasks with a large number of steps that require a different type of expertise at each level,” he said. “Working in a large consortium has obvious advantages for such projects. We are part of a well-organized project where each partner having a clear idea of his role leads to quick turnaround. Proper and clear communication is vital for the success of our project. We are using online repositories for sharing of codes as well as data or information. Weekly video conferencing allows us to make checks on progress and remain in sync, along with frequent chats between concerned subsets of people, and this has made it possible to successfully move forward in step.”
For GCS leadership, this crisis has shown that making sure that computing resources are quickly and efficiently deployed for researchers in the midst of a crisis is of the utmost importance. “At LRZ, we have discussed the need for detailed plans to address the next crisis, not necessarily a pandemic,” Kranzlmüller said. “We had an internal plan, and were able to send all staff into home office within a few days, but we also have a long tradition focusing on biological and virology research, computational climate science, and other research areas that could be relevant for future disasters or crises. We want to ensure when the next crisis comes, supercomputers are among the first technological resources capable of being deployed in support of front-line efforts.”
Lippert, who has studied both computational science and quantum physics at the PhD level and is a vocal advocate for science, remains positive due to his trust in the international scientific community.
Any vaccine will come from science, any measure to be validated for epidemiology will come from science, any pharmaceutical therapy will come from science, any understanding of the hygienic aspects needed to redesign or rebuild public places where people are gathering together, these are all things that are to be understood scientifically,” he said. “And I believe we will be successful because of the strength of science in Germany, Europe, and around the world.”